I was hit with three articles this morning that cut right to the heart of some work we’re doing on domain specific knowledgebases. AI projects require data, domain expertise, good applications developers and the right combination of AI technology.
One of the first questions we get asked is “can you handle big data, can you scale?” The answer is almost always, “yes …. but you don’t have/need big data, for this project you need high quality small data”.
The key to success is getting the right data collected, enriched and in the database first, not vacuum up the universe and try to filter out the crap (noise).
Below are some highlights from the articles by Howard Lindzon, Nassim Taleb and Shane Parrish. In our work, we are constantly faced with trying to “teach” the computer to understand the data as a human (smart but not necessarily expert) would understand it so as to extract insight and support decision making. Providing value to our clients is first understanding what we can’t do and where the system constraints impact the results, just as we need to understand bias in our own decision-making.
Howard Lindzon – Huge Wins, Small Data
First up this morning was this post by Howard Lindzon, one of my favorite bloggers, Small Data For Big Wins. In it he quotes, Nassim Taleb from WIRED. The gist:
WE’RE MORE FOOLED by noise than ever before, and it’s because of a nasty phenomenon called “big data.” With big data, researchers have brought cherry-picking to an industrial level.
Modernity provides too many variables, but too little data per variable. So the spurious relationships grow much, much faster than real information.
In other words: Big data may mean more information, but it also means more false information.
Howard on small data for investors,
Most traders and investors get bogged down with too many variables and too much information which leads to asking too many people for opinions and most likely too many positions…and on and on.
I have had huge wins using small data…the actual closing price of stocks, my eyes, my ears and following the right people.
In another post, Big Data or Small Data – Economists and Analysts Stink With Both…and it will get Worse with Social Data, he hit on the same topic from a different perspective,
Stay away from big in today’s financial world. It has not worked in the past unless you were the house. The house got bigger after 2008, but the rest of us got smaller. Embrace it.
Nassim Taleb – On Dangers of Research Based on Big Data
Nassim Taleb’s book, The Black Swan: The Impact of the Highly Improbable, is the most reread book in my library. It taught me more about how to think than any other and has been especially helpful in our AI work.
Below I pulled some excerpts from his article in WIRED, BEWARE THE BIG ERRORS OF 'BIG DATA' which draws from his new book Antifragile – Things That Gain Disorder. His point here is to understand the impact of the use of big data on research and proceed with caution despite the claims.
Just like bankers who own a free option – where they make the profits and transfer losses to others – researchers have the ability to pick whatever statistics confirm their beliefs (or show good results) ... and then ditch the rest.
Big-data researchers have the option to stop doing their research once they have the right result. In options language: The researcher gets the “upside” and truth gets the “downside.” It makes him antifragile, that is, capable of benefiting from complexity and uncertainty – and at the expense of others.
But beyond that, big data means anyone can find fake statistical relationships, since the spurious rises to the surface. This is because in large data sets, large deviations are vastly more attributable to variance (or noise) than to information (or signal). It’s a property of sampling: In real life there is no cherry-picking, but on the researcher’s computer, there is. Large deviations are likely to be bogus.
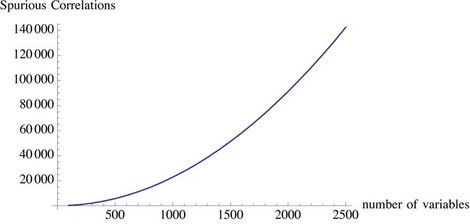
This is the tragedy of big data: The more variables, the more correlations that can show significance. Falsity also grows faster than information; it is nonlinear (convex) with respect to data (this convexity in fact resembles that of a financial option payoff). Noise is antifragile.
Big data can tell us what's wrong, not what's right.
Shane Parrish – Farnham Street and the News
Our specialty in the AI field is working with language or unstructured text. Many of our client applications revolve around teaching the machine to understand news, and while not the same “big” as social media “big”, the datasets get large. And as we are all reminded daily, its full of Fake News….
From Shane,
To me, this relates to reading the news.
We're consumed—bombarded even—by all of this incoming information that's constructed in a way to capture and maintain our attention. Somewhat counter-intuitively, this distraction offers negative, not positive utility. Not only does it give us easily accessible information that's full of noise from people that are not deeply fluent in the subject they are talking about but we rarely consider the opportunity cost of this time or the false confidence it gives us (which causes us to take undue risks).
It would be much better to focus our limited attention in two places.
The first, our niche. That is our narrow specialization or our circle of competence. This is after all how we'll make a living.
The second, on how the world works. These are the time-tested ideas that repeat throughout history and don't change as time passes. These are the mental models that you can use to not only better understand how the world works and why people behave as they do, but also to make better decisions.
They combine a narrow specialization with a general view of how the world works. If you further add how to think, now you're really getting somewhere.
“Right size” Your Data, Bigger Not Always Better
My takeaway from this trio of articles:
- Small data works for big wins in investing, don’t get lost in the chatter.
- In research, proceed with caution when the validity is buried in “huge” dark data models.
- In news, avoid distraction by staying in your domain and using your mental models as a compass.
I think this is good advice whether you’re working with market, research or news, but want you to be especially aware of how these same issues impact the applications powered by AI that are soon to be everywhere. This is a warning I’d like you to put into your mental model.